At Meadow Brooke, we don’t just sell and talk about Agentic AI — we use it to transform the way we work.
Every week in our Agent Lab, we test new ideas — not to theorise about automation, but to solve real problems in real workflows. One recurring challenge? Curating the best AI news and use cases from an ever-expanding sea of content. Our goal was to share meaningful updates with our audience. But the manual work of finding and drafting them? Time-consuming and unsustainable.
We knew there had to be a smarter, more efficient way — so we built one.
Meet Daisy 🌼, our internal AI content agent designed to help us surface relevant, high-quality AI stories for our “Daily Bites” — quickly, intelligently, and cost-effectively.

Since n8n is our go-to platform for Agentic AI experimentation, we naturally used it to bring Daisy to life.
This post walks through our first week building Daisy — what we tested, what worked (and didn’t), and what we learned about saving time, optimising costs, and getting real value from Agentic AI.
Why Daisy? Because Time (and Quality) Matter
As a fast-moving consultancy, we believe in showing our work — and improving it week by week. Content curation was a drain on time and creative energy. The team was spending hours scanning newsletters and RSS feeds just to find that one story worth sharing.
We needed an AI content agent that aligned with how we work:
- Fast iterations
- High-quality outputs
- Time and cost efficiency
- Alignment with our brand voice and goals
Daisy now powers our internal Linkedin content curation flow, helping us deliver consistent, timely, high-quality LinkedIn posts without the heavy lift. But she didn’t start out fully formed — we got there through small, smart experiments.
Flow 1: The MVP – Fast, Simple, Imperfect
The first version of Daisy was built in a single day.
We kept it intentionally simple: pull articles from selected RSS feeds, choose the top one based on a basic keyword match, and use OpenAI to generate a LinkedIn draft. No complex logic, no filters — just enough to test the full flow.
✅ Why it was the perfect starting point:
- It proved the concept. Daisy could already generate usable content.
- It built momentum. We shipped quickly and got feedback right away.
- It built trust. Seeing a working version motivated the team and sparked new ideas.
- It saved time immediately. Even this basic AI content agent shaved off 45 minutes of manual work per post.
⚠️ But:
- The article picked wasn’t always the best — it often matched a keyword without being particularly insightful or new.
Still, it was exactly what we needed: a real, working agent to build on.
Flow 2: Filter First, Analyse Second
In the second version, we aimed to scale and refine.
We wanted Daisy to pick the best article from a much broader set of sources, so we integrated additional RSS feeds. More content meant better options — but it also introduced more noise and higher token usage if we passed it all to OpenAI.
To stay lean and smart, we built a two-part upgrade:
- A custom rating logic, designed to score articles based on our editorial objectives — relevance, uniqueness, and usefulness to our audience.
- A JavaScript-based filter, which pre-processed the articles to eliminate anything clearly irrelevant before passing them to OpenAI for deeper analysis.
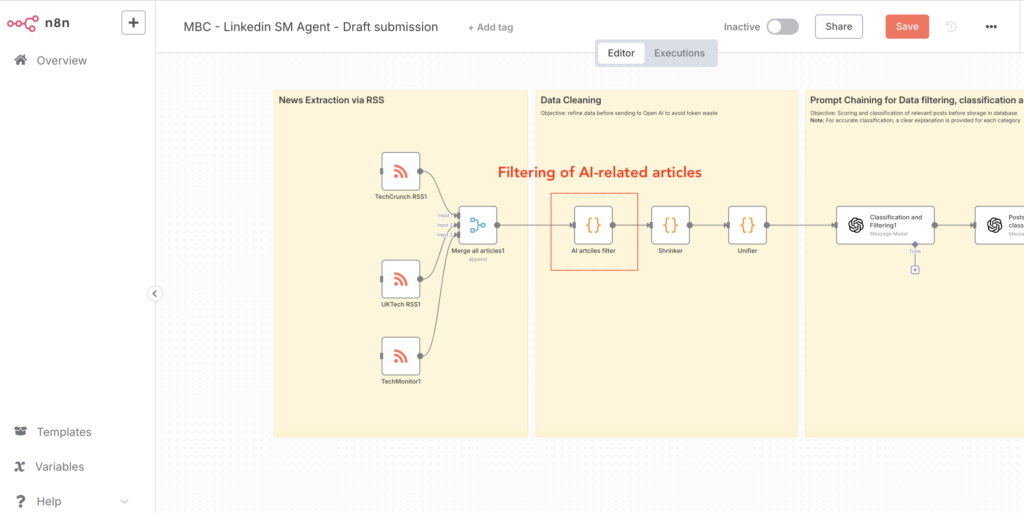
✅ What worked:
- We expanded our content pool and gave Daisy more to work with.
- Pre-filtering helped reduce token usage and improved efficiency.
- The rating logic aligned agent output with our content goals.
⚠️ But:
- The filters were too rigid. If an article didn’t use the exact terms, it was excluded — even if it was highly relevant.
- We started missing out on great content due to overly strict controls.
This taught us that cost optimisation without nuance can hurt quality, especially in areas where context matters.
Flow 3: Let OpenAI Handle It
For our third iteration, we let go of the pre-filters and leaned into OpenAI’s strength: nuanced understanding.
Instead of trying to narrow things down beforehand, we sent the entire list of articles to OpenAI and asked it to evaluate them against our criteria: insight, relevance, clarity, and alignment with our brand tone.
✅ What worked:
- The AI content agent surfaced consistently better articles — ones we often wouldn’t have selected manually.
- The content generated was sharper, more engaging, and more aligned with our themes.
- It saved us even more time — not just in research, but in confidence that the output was ready to go.
⚠️ The trade-off:
- It used more tokens — but the difference in cost was modest compared to the value gained in quality and saved hours.
Flow 3 became our go-to setup: high input, high understanding, and high payoff.
What to learn from Daisy’s first week
- Start with an MVP. It’s not just about proving tech — it builds momentum, trust, and real insight into what works.
- Saving tokens isn’t always worth it. Don’t compromise quality just to reduce cost — look at the full ROI, including time saved.
- Smart experiments lead to smart systems. Iterating through flows helped us find a balance of speed, cost, and content quality.
- Agents aren’t just for big, complex tasks. Daisy supports a daily task — and that’s where the compounding value lies.
Want to See Daisy in Action?
Whether you’re a tech leader exploring AI integration, or a marketing leader looking for consistent, high-quality content without the grind — Daisy shows what’s possible when you combine Agentic AI with real-world needs.
If you’re curious how something like this could work in your world —
👋 Contact us. We’d love to show you.